
"No problem can be solved from the same level of consciousness that created it"
– Albert Einstein
What is Deepfake?
Tech companies like Facebook, Microsoft, and Google are facing problems dealing with the onslaught of AI-generated fake media known as Deepfakes. “Deepfake” is so named because it uses Deep Learning (DL) technology – a branch of Machine Learning that applies net simulation to massive data sets, to create a “FAKE”. Deepfake videos or images are created by replacing a face in an existing image or video with a fake mask produced using AI.
What is Deep Learning?
Deep Learning is a part of Machine Learning (ML) based on Artificial Neural Networks (ANNs). Learning can be supervised, semi-supervised or unsupervised. ANN is a powerful model in ML inspired by the computation and communication capabilities of the brain. An ANN is based on a collection of connected units or nodes called artificial neurons, which model the neurons in a biological brain. Each connection, like the synapses in a biological brain, can transmit a signal to other neurons. An artificial neuron receives a signal, then processes it and signal neurons connected to it.
ANN has two main components – Synapse and Neuron.
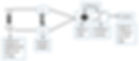
A Neuron-Synapse model is shown above in which inputs from neighbouring neurons are summed using the synaptic weights, and a nonlinear activation function determines the output of the neuron. The model is implemented using digital-like logic using spikes. A neuristor is a device that can capture the essential property of a neuron – that is, the ability to generate a spike or impulse of activity when some threshold is exceeded. A neuristor uses a relatively simple electronic circuit to generate spikes. Incoming signals charge a capacitor that is placed in parallel with a device called a memristor. The memristor behaves like a resistor except that once the small currents passing through it start to heat it up, its resistance rapidly drops off. The charge built up on the capacitor by incoming spikes discharges, - a spiking neuron comprised of just two elementary circuit elements.
Deepfakes Uses ML Techniques known as Autoencoder and Generative Adversarial Networks (GANs)
An autoencoder is an artificial neural network that learns to copy its input to its output with internal (hidden) layers comprising a code. The input is mapped into the code by an encoder and the code is reconstructed to the original input by a decoder. The autoencoder can be used for denoising or noise reduction from a signal (which can be an image, audio or a document). The autoencoder can be trained using Feedforward, Long Short-Term Memory (LSTM), and/or Convolution neural network. In contrast to autoencoders, Generative Adversarial Networks (GANs) are able to produce/generate new content using generative models. GANs architecture includes a generator and a discriminator. The generator is a neural network that models a transform function (new data instances) while the discriminator, evaluates and/or decides whether each instance of data that it reviews belongs to the actual training data set or not.
Here are the steps a GAN takes:
The generator takes in random numbers and returns an image.
This generated image is fed into the discriminator alongside a stream of images taken from the actual dataset.
The discriminator takes in both real and fake images and returns probabilities, a number between 0 and 1, with 1 representing a prediction of authenticity and 0 representing fake.
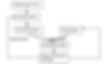
Deepfake technology has positive applications in many industries including movies, educational media, games, and entertainment, social media and healthcare, material science and various business fields such as fashion and e-commerce.
1. The film industry utilizes Deepfake technology in multiple ways. It is used in making digital voices for actors who lost theirs due to disease, updating film footage instead of reshooting it, recreating classic scenes in movies, creating new movies starring dead actors and improving the quality of videos. Deepfake technology also allows for voice dubbing for movies in any language, thus allowing diverse audiences to better enjoy films and educational media. Similarly, Deepfake technology can break the language barrier on video conference calls by translating speech and simultaneously changing facial movements to improve eye contact and make everyone appear to be speaking the same language.
2. The technology behind Deepfakes also enables multiplayer games and virtual chat worlds to be more real-looking. This helps to develop better human relationships and interactions online. Similarly, technology has positive uses in the medical and social fields as well. It can digitally recreate an amputee's limb or allow transgender people to better see themselves as a preferred gender. Moreover, GANs (Generative Adversarial Networks) which are used to produce Deepfake content can also be used to detect abnormalities in X-rays and in creating virtual chemical molecules to speed up materials science and medical discoveries.
3. Businesses can utilize Deepfake technology to transform e-commerce and advertising in significant ways. For example, brands can show fashion outfits on a variety of models with different skin tones, heights, and weights by deepfaking the facial features of the model. Deepfakes can turn consumers themselves into models by enabling the virtual fitting to preview how an outfit would look on them before purchasing. Further, this technology allows people to create digital clones of themselves and virtually experience a place such as a wedding venue.
Deepfakes have the potential to overcome the technological loopholes in smart assistants. The actions of virtual humans can't be pre-programmed in a traditional hard-coded sense. Thus, Deepfake tech typically takes tons of examples of human behaviour as inputs and then produces outputs that approximate that behaviour. It provides smart assistants with the capacity and flexibility to understand and originate conversations with much more sophistication.
On the other hand, the same technology has nefarious applications aplenty as well:
1) More pressure on journalists struggling to filter real from fake news:
Deepfakes pose a greater threat than fake news because they are harder to spot and people are inclined to believe the fake is real. The technology allows the production of seemingly legitimate news videos that place the reputation of journalists and the media at risk.
2) Threatens national security:
The intelligence communities worldwide are concerned about the use of Deepfaked content to spread political propaganda and disrupt election campaigns in their nations. A foreign intelligence agency could produce a Deepfake video of a politician using a racist comment or taking a bribe, a presidential candidate confessing complicity in a crime, or warning another country of an upcoming war or admitting a secret plan to carry out a conspiracy or soldiers committing war crimes. While such faked videos would likely cause domestic unrest, riots, and disruptions in elections, other nations could even choose to develop their foreign policies based on this fake content leading to international conflicts.
3) Hampers citizen trust toward information by authorities:
Deepfakes hampers digital literacy and citizens' trust in authority-provided information. The most damaging aspect of Deepfakes may not be disinformation, but rather how constant contact with misinformation leads people to feel that information simply cannot be trusted and thereby resulting in a phenomenon known as "information apocalypse". Indeed, people nowadays are increasingly affected by AI-generated spam, and by fake news that builds on biased text, faked videos, and loads of conspiracy theories.
4) Raises cybersecurity issues for people and organizations:
Cybersecurity issues constitute another threat imposed by Deepfakes. Deepfakes could be used for market and stock manipulation, for example, by showing a chief executive saying racist or misogynistic slurs, announcing a fake merger, making false statements of financial losses or bankruptcy, or portraying them as if committing a crime. Deepfaked porn or product announcements could be used for brand sabotage, blackmail or embarrass management. Further, Deepfake technology can create a fraudulent identity and convert an adult face into a child's or younger person's face, raising concerns about the use of technology by child predators. Lastly, Deepfakes can contribute to the spread of malicious scripts. Recently, researchers found that a website devoted to Deepfakes used its visitor's computers to mine cryptocurrencies.
Methods of Prevention
1) Legislation and regulation:
Deepfakes are not specifically addressed by civil or criminal laws. Legal experts have suggested adapting current laws to cover defamation, identity fraud, or impersonating a government official using Deepfakes but the increasing use of AI technologies calls for new laws and regulatory frameworks. Thus, regulators must develop difficult laws around free-speech and ownership laws to properly regulate the use of Deepfake technology.
2) Corporate policies and voluntary action:
Corporates and public figures can themselves take some voluntary action against Deepfakes. Politicians can commit not to use illicit digital campaign tactics or spread disinformation such as Deepfakes in their election campaigns. Social media companies should collaborate to prevent their platforms from being used for disinformation and proactively enforce policies to block and remove Deepfakes. Presently, many companies do not remove disputed content but down rank it to make it more difficult to find. On the other hand, some firms take more action, such as suspending user accounts and investing in quicker detection technology. Facebook cuts off any content identified as false or misleading by third-party fact-checkers from running ads and making money. Instagram's algorithms do not recommend people view content that is marked false by fact-checkers. Among news media companies, Wall Street Journal and Reuters have formed corporate teams to help and train their reporters to identify fake content and to adopt detection techniques and tools such as cross-referencing locations on Google maps and reverse image searching.
3) Education and training:
There is a need to raise public awareness about AI's potential for misuse. Governments, regulators, and individuals need to understand that a video may not provide an accurate representation of what happened. It is recommended that critical thinking and digital literacy be taught in schools as these traits contribute to children's ability to spot fake news and interact more respectfully with each other online. These skills should also be promoted among the older less tech-savvy population.
4) Anti-Deepfake technology:
Anti-Deepfake technology can be utilized to detect Deepfakes, authenticate content and prevent content from being used to produce Deepfakes. The fact that there are far more available research resources and people working on developing technology to create Deepfakes than to detect the technology creates a massive challenge. For instance, researchers found that early Deepfake methods can be detected by analyzing the rate of blinking. However, recent Deepfake videos have fixed the lack of blinking after the findings were published. Nonetheless, Media forensic experts have suggested subtle indicators to detect
Deepfakes, including a range of imperfections such as face wobble, shimmer, and distortion; inconsistencies with speech and mouth movements; abnormal movements of fixed objects such as a microphone stand; inconsistencies in lighting, reflections and shadows; blurred edges; angles and blurring of facial features; lack of breathing; unnatural eye direction; missing facial features such as a known mole on a cheek; softness and weight of clothing and hair; overly smooth skin; missing hair and teeth details; misalignment in face symmetry; inconsistencies in pixel levels; and strange behaviour of an individual doing something implausible. Further, AI algorithms can analyze imperfections unique to the light sensor of specific camera models that can detect subtle changes occurring on a person's face in a video. New fake-detection algorithms based on mammalian auditory systems can either look at videos on a frame-by-frame basis to track signs of forgery or review the entire video at once to examine soft biometric signatures, including inconsistencies in the authenticated relationships between head movements, speech patterns, and facial expressions such as smiling to determine if the video has been manipulated.
The problem with Deepfakes is not only about proving something is false, but also about proving that an object is authentic. Digital watermarks can be used to authenticate content by creating a digital watermark at the moment of a film's recording. Upon footage playback, its watermark can be compared with the original fingerprint to check for a match, and provide the viewer with a score that indicates the likelihood of tampering. Video authenticity is also provided by mapping its origin and how it has traveled online. Blockchain technology can help in verifying the origins and distribution of videos by creating and storing digital signatures in a ledger that is almost impossible to manipulate. Attestiv Inc., a Massachusetts based company, utilizes similar blockchain technology to assure the authenticity of digital media.
Seminla Patent Applications
Attestiv Inc. has filed a couple of patent applications (US20190391972A1 & US20200012806A1) in 2019. These applications propose to store the metadata of digital content (images or videos) on an immutable distributed ledger, for instance, a blockchain. The metadata of content includes identifiers or fingerprints. In order to determine the authenticity of digital content, the fingerprint corresponding to that digital content is determined. Then, the fingerprint is mapped to an address within the immutable distributed ledger to retrieve metadata. The retrieved metadata contains the original fingerprint which is then compared with the determined fingerprint for validation of content.

Another method to prevent the creation of Deepfakes is inserting noise into photos or videos. The added noise is not visible to the human eye but it prevents the visual content from being used by Deepfake software. Specifically designed 3D-printed glasses can also be used to trick Deepfake software into misclassifying the wearer. This technology could help likely targets such as politicians, celebrities and executives to prevent Deepfakes being made of them. Also, researchers who are developing GAN technologies can design and put proper guidelines in place so that their technologies become more difficult to misuse for disinformation purposes.
Conclusion
Deepfake videos have significant implications and hence require the attention of both government and corporates. Yet the social networking industry doesn't have a great data set or benchmark for detecting them. Thus, Facebook partnered with Microsoft and Amazon to build the Deepfake Detection Challenge (DFDC). The challenge includes a data set, leader board, as well as compensation to create new ways of detecting and preventing media manipulated via AI from being used to mislead others. Google also released a large dataset of visual Deepfakes to initiate a similar international challenge. However, it seems that these tech giants are more interested in outsourcing the issue rather than taking it into their own hands and investing directly to find the solution. #artificialintelligence #emergingtech #patents #technology
Keywords: ai, artificial intelligence, ai technology, ai companies, artificial intelligence examples, future of ai, artificial intelligence future, artificial intelligence companies, ai software, ai ml, deepfake ai, deepfake, deepfake technology, deepfake examples, best deepfakes, deep learning, neural network, deepfake videos, deepfake meaning, deepfake software, deepfake maker